共计 5325 个字符,预计需要花费 14 分钟才能阅读完成。
Harris角点
基本原理
人眼对角点的识别通常是在一个局部的小区域或小窗口完成的。如果在各个方向上移动这个特征的小窗口,窗口内区域的灰度发生了较大的变化,那么就认为在窗口内遇到了角点。如果这个特定的窗口在图像各个方向上移动时,窗口内图像的灰度没有发生变化,那么窗口内就不存在角点;如果窗口在某一个方向移动时,窗口内图像的灰度发生了较大的变化,而在另一些方向上没有发生变化,那么,窗口内的图像可能就是一条直线的线段。
对于图像I(x,y),当在点(x,y)处平移(Δx,Δy)后的自相似性,可以通过自相关函数给出:
其中,W(x,y)是以点(x,y)为中心的窗口,w(u,v)为加权函数,它既可是常数,也可以是高斯加权函数。
根据泰勒展开,对图像I(x,y)在平移(Δx,Δy)后进行一阶近似:
其中,Ix,Iy是图像I(x,y)的偏导数,这样的话,自相关函数则可以简化为:
其中
也就是说图像I(x,y)在点(x,y)处平移(Δx,Δy)后的自相关函数可以近似为二项函数:
其中:
二次项函数本质上就是一个椭圆函数。椭圆的扁率和尺寸是由M(x,y)的特征值λ1、λ2决定的,椭贺的方向是由M(x,y)的特征矢量决定的,如下图所示,椭圆方程为:
椭圆函数特征值与图像中的角点、直线(边缘)和平面之间的关系如下图所示。共可分为三种情况:
– 图像中的直线。一个特征值大,另一个特征值小,λ1≫λ2或λ2≫λ1。自相关函数值在某一方向上大,在其他方向上小。
– 图像中的平面。两个特征值都小,且近似相等;自相关函数数值在各个方向上都小。
– 图像中的角点。两个特征值都大,且近似相等,自相关函数在所有方向都增大。
根据二次项函数特征值的计算公式,我们可以求M(x,y)矩阵的特征值。但是Harris给出的角点差别方法并不需要计算具体的特征值,而是计算一个角点响应值R来判断角点。R的计算公式为:
式中,detM为矩阵M=[ABBC]的行列式;traceM为矩阵M的直迹;α为经常常数,取值范围为0.04~0.06。事实上,特征是隐含在detM和traceM中,因为:
说明
– 图像(x,y)与平移(Δx,Δy)之后得到的点之间的自相似性可以使用自相关函数表示。自相关函数维基百科上给出的定义如下:
根据期望的定义可以分解为上述像素点之间的近似性描述。
– R的值决定了该像素点是否为角点。根据矩阵理论特征值与聚珍版本身的联系,R值的求解至于特征值本身存在着关系。当特征值都大时,R值也随之变大。特征值都小时,R值更小。特征之一大一小,结果也不会比之前描述的情况大,这介于特征值都大和特征值都小之间。
Harris算法流程
根据上述讨论,可以将Harris图像角点检测算法归纳如下,共分以下五步:
– 计算图像I(x,y)在X和Y两个方向的梯度Ix、Iy。
– 计算图像两个方向梯度的乘积。
– 使用高斯函数对I2x、I2y和Ixy进行高斯加权(取σ=1),生成矩阵M的元素A、B和C。
– 计算每个像素的Harris响应值R,并对小于某一阈值t的R置为零。
– 在3×3或5×5的邻域内进行非最大值抑制,局部最大值点即为图像中的角点。
实验数据分析
//HarrisDect.h
#if !defined HARRISD
#define HARRISD
#include <vector>
#include <opencv2/core/core.hpp>
#include <opencv2/imgproc/imgproc.hpp>
#include <opencv2/highgui/highgui.hpp>
#include <opencv2/features2d/features2d.hpp>
#include <opencv2/nonfree/features2d.hpp>
class HarrisDetector {
private:
// 32-bit float image of corner strength
cv::Mat cornerStrength;
// 32-bit float image of thresholded corners
cv::Mat cornerTh;
// image of local maxima (internal)
cv::Mat localMax;
// size of neighbourhood for derivatives smoothing
int neighbourhood;
// aperture for gradient computation
int aperture;
// Harris parameter
double k;
// maximum strength for threshold computation
double maxStrength;
// calculated threshold (internal)
double threshold;
// size of neighbourhood for non-max suppression
int nonMaxSize;
// kernel for non-max suppression
cv::Mat kernel;
public:
HarrisDetector() : neighbourhood(3), aperture(3), k(0.1), maxStrength(0.0), threshold(0.01), nonMaxSize(3) {
setLocalMaxWindowSize(nonMaxSize);
}
// Create kernel used in non-maxima suppression
void setLocalMaxWindowSize(int size) {
nonMaxSize= size;
kernel.create(nonMaxSize,nonMaxSize,CV_8U);
}
// Compute Harris corners
void detect(const cv::Mat& image) {
// Harris computation
cv::cornerHarris(image,cornerStrength,
neighbourhood,// neighborhood size
aperture, // aperture size
k); // Harris parameter
// internal threshold computation
double minStrength; // not used
cv::minMaxLoc(cornerStrength,&minStrength,&maxStrength);
// local maxima detection
cv::Mat dilated; // temporary image
cv::dilate(cornerStrength,dilated,cv::Mat());
cv::compare(cornerStrength,dilated,localMax,cv::CMP_EQ);
}
// Get the corner map from the computed Harris values
cv::Mat getCornerMap(double qualityLevel) {
cv::Mat cornerMap;
// thresholding the corner strength
threshold= qualityLevel*maxStrength;
cv::threshold(cornerStrength,cornerTh,threshold,255,cv::THRESH_BINARY);
// convert to 8-bit image
cornerTh.convertTo(cornerMap,CV_8U);
// non-maxima suppression
cv::bitwise_and(cornerMap,localMax,cornerMap);
return cornerMap;
}
// Get the feature points vector from the computed Harris values
void getCorners(std::vector<cv::Point> &points, double qualityLevel) {
// Get the corner map
cv::Mat cornerMap= getCornerMap(qualityLevel);
// Get the corners
getCorners(points, cornerMap);
}
// Get the feature points vector from the computed corner map
void getCorners(std::vector<cv::Point> &points, const cv::Mat& cornerMap) {
// Iterate over the pixels to obtain all feature points
for( int y = 0; y < cornerMap.rows; y++ ) {
const uchar* rowPtr = cornerMap.ptr<uchar>(y);
for( int x = 0; x < cornerMap.cols; x++ ) {
// if it is a feature point
if (rowPtr[x]) {
points.push_back(cv::Point(x,y));
}
}
}
}
// Draw circles at feature point locations on an image
void drawOnImage(cv::Mat &image, const std::vector<cv::Point> &points, cv::Scalar color= cv::Scalar(255,255,255), int radius=3, int thickness=2) {
std::vector<cv::Point>::const_iterator it= points.begin();
// for all corners
while (it!=points.end()) {
// draw a circle at each corner location
cv::circle(image,*it,radius,color,thickness);
++it;
}
}
};
#endif
主程序:
#include <iostream>
#include <vector>
#include <HarrisDect.h>
int main()
{
// Read input image
cv::Mat image= cv::imread("church01.jpg",0);
if (!image.data)
return 0;
// Display the image
cv::namedWindow("Original Image");
cv::imshow("Original Image",image);
// Detect Harris Corners
cv::Mat cornerStrength;
cv::cornerHarris(image,cornerStrength,
3, // neighborhood size
3, // aperture size
0.01); // Harris parameter
// threshold the corner strengths
cv::Mat harrisCorners;
double threshold= 0.0001;
cv::threshold(cornerStrength,harrisCorners,
threshold,255,cv::THRESH_BINARY_INV);
// Display the corners
cv::namedWindow("Harris Corner Map");
cv::imshow("Harris Corner Map",harrisCorners);
// Create Harris detector instance
HarrisDetector harris;
// Compute Harris values
harris.detect(image);
// Detect Harris corners
std::vector<cv::Point> pts;
harris.getCorners(pts,0.01);
// Draw Harris corners
harris.drawOnImage(image,pts);
// Display the corners
cv::namedWindow("Harris Corners");
cv::imshow("Harris Corners",image);
cv::waitKey();
}
注释:实验代码借用Opencv2计算机视觉编程手册,发现作者给出的代码出现部分头文件缺失,代码地址为[点击这里](https://github.com/ITpublishing/opencv-2-cookbook-src)
只要在头文件中加入#include <opencv2/nonfree/features2d.hpp>即可!
## 结果示意图 ##
实验原图:
角点图:
原图+角点:
结果分析
对于实验的结果图中检测出大量的角点,但是通过改变角点响应值的阈值大小可以同步改变被检测出角点的数量。
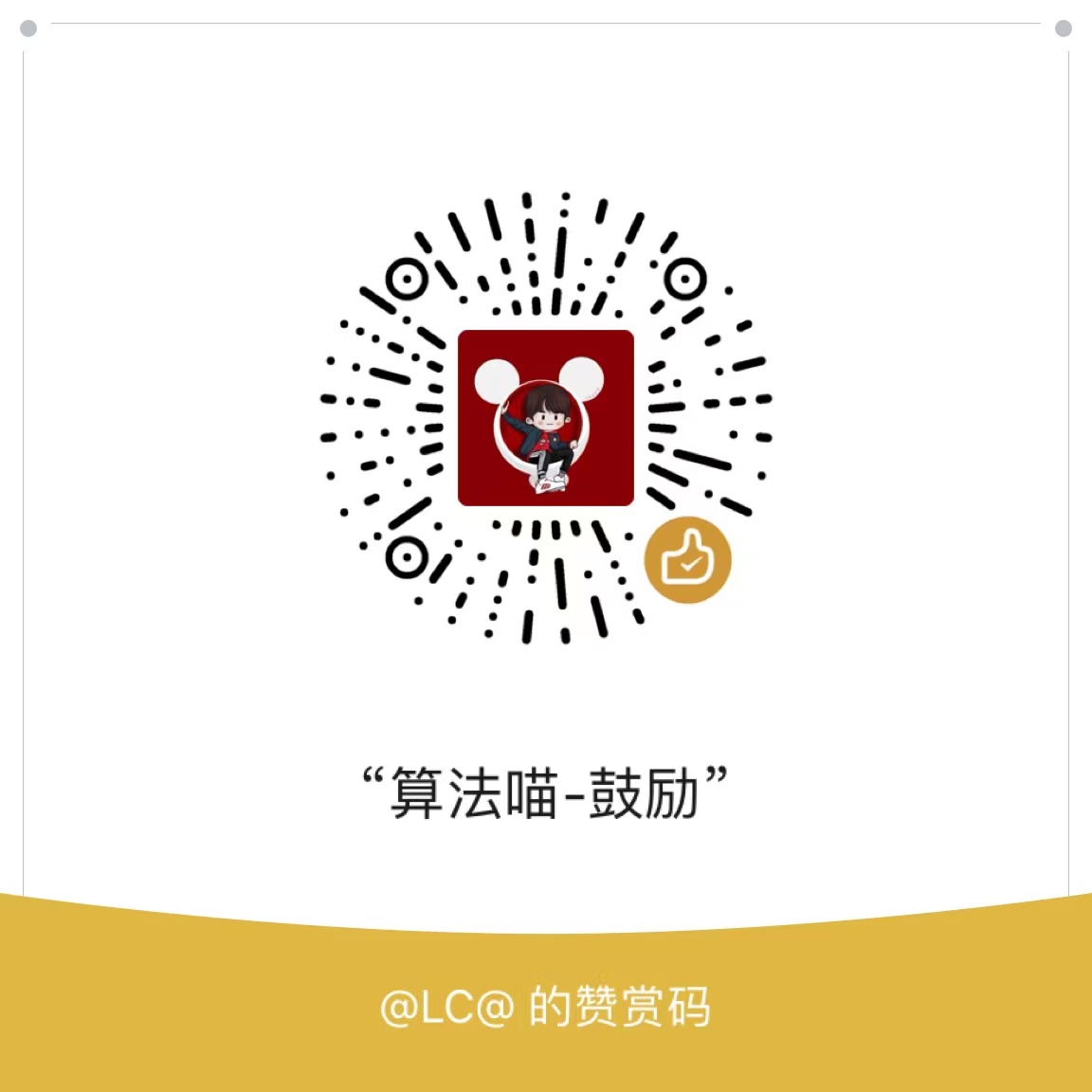