共计 4549 个字符,预计需要花费 12 分钟才能阅读完成。
最近在看一些图像去重的一些方法,网上一搜就会看到phash、dhash和ahash等基于哈希方法的去重算法,这一点跟文本上的simhash和minhash有着“相似”的处理逻辑。
phash具体处理逻辑如下所示:
- 缩小尺寸 为了后边的步骤计算简单些
- 简化色彩 将图片转化成灰度图像,进一步简化计算量
- 计算DCT 计算图片的DCT变换,得到32*32的DCT系数矩阵。
- 缩小DCT 虽然DCT的结果是32*32大小的矩阵,但我们只要保留左上角的8*8的矩阵,这部分呈现了图片中的最低频率。
- 计算平均值 如同均值哈希一样,计算DCT的均值。
- 计算hash值 根据8*8的DCT矩阵,设置0或1的64位的hash值,大于等于DCT均值的设为”1”,小于DCT均值的设为“0”。组合在一起,就构成了一个64位的整数,这就是这张图片的指纹。
其他的hash方法有着类似的处理逻辑,代码实现有很多了,google一下出来了。
基于预训练模型
使用这种方法实践应该是最快的,只要调用一下预训练model,然后获取输出即可,常见的像vgg/resnet/mobilenet等预训练网络,这些网络在超大的ImageNet上面都有过训练。本质上还是使用卷积网络不断的提取特征的过程,像vgg16动辄上亿的参数,真的是很大。
说到预训练在图像和nlp都是有着很大的意义,之前研究过bert预训练模型也是很强大,这个可以帮助下游任务提升效果。
目前keras和tensorflow里面都自带了很多预训练模型
from keras.applications.vgg16 import VGG16
from keras.preprocessing import image
from keras.applications.vgg16 import preprocess_input
import numpy as np
import os
import tensorflow as tf
os.environ["CUDA_VISIBLE_DEVICES"] = "6"
gpu_options = tf.GPUOptions(allow_growth=True)
sess = tf.Session(config=tf.ConfigProto(gpu_options=gpu_options))
from keras.utils import plot_model
from matplotlib import pyplot as plt
#【0】VGG16模型,加载预训练权重,不保留顶层的三个全连接层
model = VGG16(weights='imagenet', include_top=False)
print(model.summary()) # 打印模型概况
plot_model(model,to_file = 'a simple convnet.png') # 画出模型结构图,并保存成图片
'''
Layer (type) Output Shape Param #
=================================================================
input_1 (InputLayer) (None, None, None, 3) 0
_________________________________________________________________
block1_conv1 (Conv2D) (None, None, None, 64) 1792
_________________________________________________________________
block1_conv2 (Conv2D) (None, None, None, 64) 36928
_________________________________________________________________
block1_pool (MaxPooling2D) (None, None, None, 64) 0
_________________________________________________________________
block2_conv1 (Conv2D) (None, None, None, 128) 73856
_________________________________________________________________
block2_conv2 (Conv2D) (None, None, None, 128) 147584
_________________________________________________________________
block2_pool (MaxPooling2D) (None, None, None, 128) 0
_________________________________________________________________
block3_conv1 (Conv2D) (None, None, None, 256) 295168
_________________________________________________________________
block3_conv2 (Conv2D) (None, None, None, 256) 590080
_________________________________________________________________
block3_conv3 (Conv2D) (None, None, None, 256) 590080
_________________________________________________________________
block3_pool (MaxPooling2D) (None, None, None, 256) 0
_________________________________________________________________
block4_conv1 (Conv2D) (None, None, None, 512) 1180160
_________________________________________________________________
block4_conv2 (Conv2D) (None, None, None, 512) 2359808
_________________________________________________________________
block4_conv3 (Conv2D) (None, None, None, 512) 2359808
_________________________________________________________________
block4_pool (MaxPooling2D) (None, None, None, 512) 0
_________________________________________________________________
block5_conv1 (Conv2D) (None, None, None, 512) 2359808
_________________________________________________________________
block5_conv2 (Conv2D) (None, None, None, 512) 2359808
_________________________________________________________________
block5_conv3 (Conv2D) (None, None, None, 512) 2359808
_________________________________________________________________
block5_pool (MaxPooling2D) (None, None, None, 512) 0
=================================================================
Total params: 14,714,688
Trainable params: 14,714,688
Non-trainable params: 0
_________________________________________________________________
'''
#【1】从网上下载一张图片,保存在当前路径下
img_path = './elephant.jpg'
img = image.load_img(img_path, target_size=(224, 224)) # 加载图片并resize成224x224
#【2】显示图片
plt.imshow(img)
plt.show()
#【3】将图片转化为4d tensor形式
x = image.img_to_array(img) # x.shape: (224, 224, 3)
x = np.expand_dims(x, axis=0) # x.shape: (1, 224, 224, 3)
#【4】数据预处理
x = preprocess_input(x) #去均值中心化,preprocess_input函数详细功能见注释
#【5】提取特征
features = model.predict(x)
print(features.shape) #(1,7,7,512)
上面的代码是参考了https://www.jianshu.com/p/568168ad4950 这篇文章的实现,不过它加载的预训练模型不包含输出层,如果你将参数include_top=True
则会发现多三层出来
model = VGG16(weights='imagenet', include_top=True)
主要是新增了fc1 fc2 softmax ,我是使用了fc2作为结果的输出表征图像的特征。
如何获取fc2输出的结果呢,这个就是keras获取中间层输出哈,
from keras import backend as K
# with a Sequential model
get_3rd_layer_output = K.function([model.layers[0].input],
[model.layers[3].output])
layer_output = get_3rd_layer_output([x])[0]
你想获取fc2层的输出可以做下修改
get_3rd_layer_output = K.function([model.layers[0].input], [model.get_layer(“fc2”).output])
最后layer_output就是你想要的fc2层输出了。
AE自编码
未完待续,先出门,回来再补上
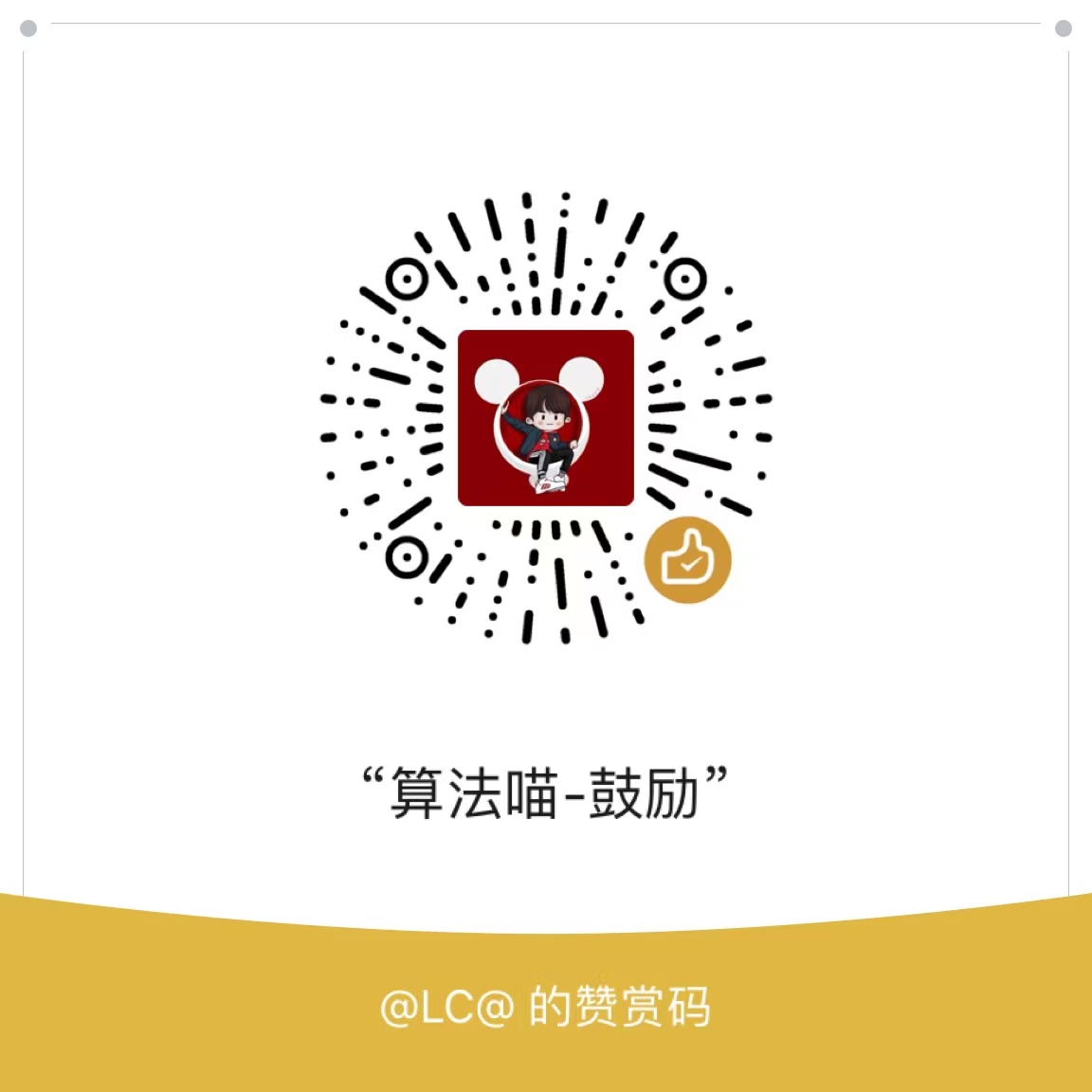