共计 3862 个字符,预计需要花费 10 分钟才能阅读完成。
上一篇讲解了使用基于递归消除法,从大范围来讲是通过wrapper的方法,中文就是包装的方法,递归消除是这其中主要的方法,还有其他类似GA等启发式搜索方法。从根本上来说基于wrapper是一种搜索方式,将当前的特征组合作为待搜索的大集合,然后在其中找出最优的特征组合然后返回结果。
区别于wrapper方法,embedded方法是在选定模型的情况下,选取出对模型训练有利的特征,常见的有L1,L2,基于L2的回归又叫岭回归
为什么说l1和l2可以用来进行特征选择?
去网上一搜给出的结果都是l1可以得到稀疏解之类的说法,的确是这种情况,现在只是用白话的方式描述一下。
最早接触l1和l2是在优化问题中的惩罚项,常见的比如线性回归如果加入l1惩罚项可以得到稀疏解,这些稀疏解就是我们求解的线性模型的各个特征的权重系数,所谓稀疏也就是说其中有些权重系数的值是为0,此时可以看到这些权重系数的为0的特征对最终的结果无贡献,那样的话就可以把这些特征去除掉,到这一步是不是就可以知道为什么l1可以用来进行特征选择。
虽然l1做到了稀疏解,但是他选出的结果并不一定是最优,他只是把具有相同贡献的特征选取其中一个作为输出的结果,没有被选中的不代表不重要,因此可以使用l2来弥补这其中的不足,具体操作为:若一个特征在L1中的权值为1,选择在L2中权值差别不大且在L1中权值为0的特征构成同类集合,将这一集合中的特征平分L1中的权值。
sklearn相关介绍
Linear models penalized with the L1 norm have sparse solutions: many of their estimated coefficients are zero. When the goal is to reduce the dimensionality of the data to use with another classifier, they can be used along with feature_selection.SelectFromModel to select the non-zero coefficients. In particular, sparse estimators useful for this purpose are the linear_model.Lasso for regression, and of linear_model.LogisticRegression and svm.LinearSVC for classification:
上述红字的部分:使用l1正则项主要是lasso和lr、svc。
svc剖析
from sklearn.feature_selection import SelectFromModel
from sklearn.svm import LinearSVC
mod=LinearSVC(C=0.01, penalty="l1", dual=False).fit(irisdata.data,irisdata.target)
selectmod=SelectFromModel(mod, prefit=True)
selectmod.transform(irisdata.data)
输出
array([[ 5.1, 3.5, 1.4],
[ 4.9, 3. , 1.4],
[ 4.7, 3.2, 1.3],
[ 4.6, 3.1, 1.5],
[ 5. , 3.6, 1.4],
[ 5.4, 3.9, 1.7],
[ 4.6, 3.4, 1.4],
[ 5. , 3.4, 1.5],
[ 4.4, 2.9, 1.4],
lasso剖析
from sklearn.linear_model import LassoCV
lassomodel=LassoCV()
selectmod1=SelectFromModel(lassomodel,threshold=0.1)
selectmod1.fit(irisdata.data,irisdata.target)
selectmod1.transform(irisdata.data)
输出
array([[ 1.4, 0.2],
[ 1.4, 0.2],
[ 1.3, 0.2],
[ 1.5, 0.2],
[ 1.4, 0.2],
[ 1.7, 0.4],
[ 1.4, 0.3],
[ 1.5, 0.2],
lr剖析
from sklearn.linear_model import LogisticRegressionCV
lrmodel=LogisticRegressionCV(penalty='l1',solver='liblinear')
selectmod2=SelectFromModel(lrmodel,threshold=10)
selectmod2.fit(irisdata.data,irisdata.target)
selectmod2.transform(irisdata.data)
输出
array([[ 1.4, 0.2],
[ 1.4, 0.2],
[ 1.3, 0.2],
[ 1.5, 0.2],
[ 1.4, 0.2],
[ 1.7, 0.4],
[ 1.4, 0.3],
[ 1.5, 0.2],
[ 1.4, 0.2],
[ 1.5, 0.1],
[ 1.5, 0.2],
使用l1和l2综合选取特征
# -*- coding: utf-8 -*-
from sklearn.linear_model import LogisticRegression
from sklearn.datasets import load_iris
from sklearn.feature_selection import SelectFromModel
class LR(LogisticRegression):
def __init__(self, threshold=0.01, dual=False, tol=1e-4, C=1.0,
fit_intercept=True, intercept_scaling=1, class_weight=None,
random_state=None, solver='liblinear', max_iter=100,
multi_class='ovr', verbose=0, warm_start=False, n_jobs=1):
#权值相近的阈值
self.threshold = threshold
LogisticRegression.__init__(self, penalty='l1', dual=dual, tol=tol, C=C,
fit_intercept=fit_intercept, intercept_scaling=intercept_scaling, class_weight=class_weight,
random_state=random_state, solver=solver, max_iter=max_iter,
multi_class=multi_class, verbose=verbose, warm_start=warm_start, n_jobs=n_jobs)
#使用同样的参数创建L2逻辑回归
self.l2 = LogisticRegression(penalty='l2', dual=dual, tol=tol, C=C, fit_intercept=fit_intercept, intercept_scaling=intercept_scaling, class_weight = class_weight, random_state=random_state, solver=solver, max_iter=max_iter, multi_class=multi_class, verbose=verbose, warm_start=warm_start, n_jobs=n_jobs)
def fit(self, X, y, sample_weight=None):
#训练L1逻辑回归
super(LR, self).fit(X, y, sample_weight=sample_weight)
self.coef_old_ = self.coef_.copy()
#训练L2逻辑回归
self.l2.fit(X, y, sample_weight=sample_weight)
cntOfRow, cntOfCol = self.coef_.shape
#权值系数矩阵的行数对应目标值的种类数目
for i in range(cntOfRow):
for j in range(cntOfCol):
coef = self.coef_[i][j]
#L1逻辑回归的权值系数不为0
if coef != 0:
idx = [j]
#对应在L2逻辑回归中的权值系数
coef1 = self.l2.coef_[i][j]
for k in range(cntOfCol):
coef2 = self.l2.coef_[i][k]
#在L2逻辑回归中,权值系数之差小于设定的阈值,且在L1中对应的权值为0
if abs(coef1-coef2) < self.threshold and j != k and self.coef_[i][k] == 0:
idx.append(k)
#计算这一类特征的权值系数均值
mean = coef / len(idx)
self.coef_[i][idx] = mean
return self
def main():
iris=load_iris()
print SelectFromModel(LR(threshold=0.5,C=0.1),threshold=1).fit_transform(iris.data,iris.target)
if __name__ == '__main__':
main()
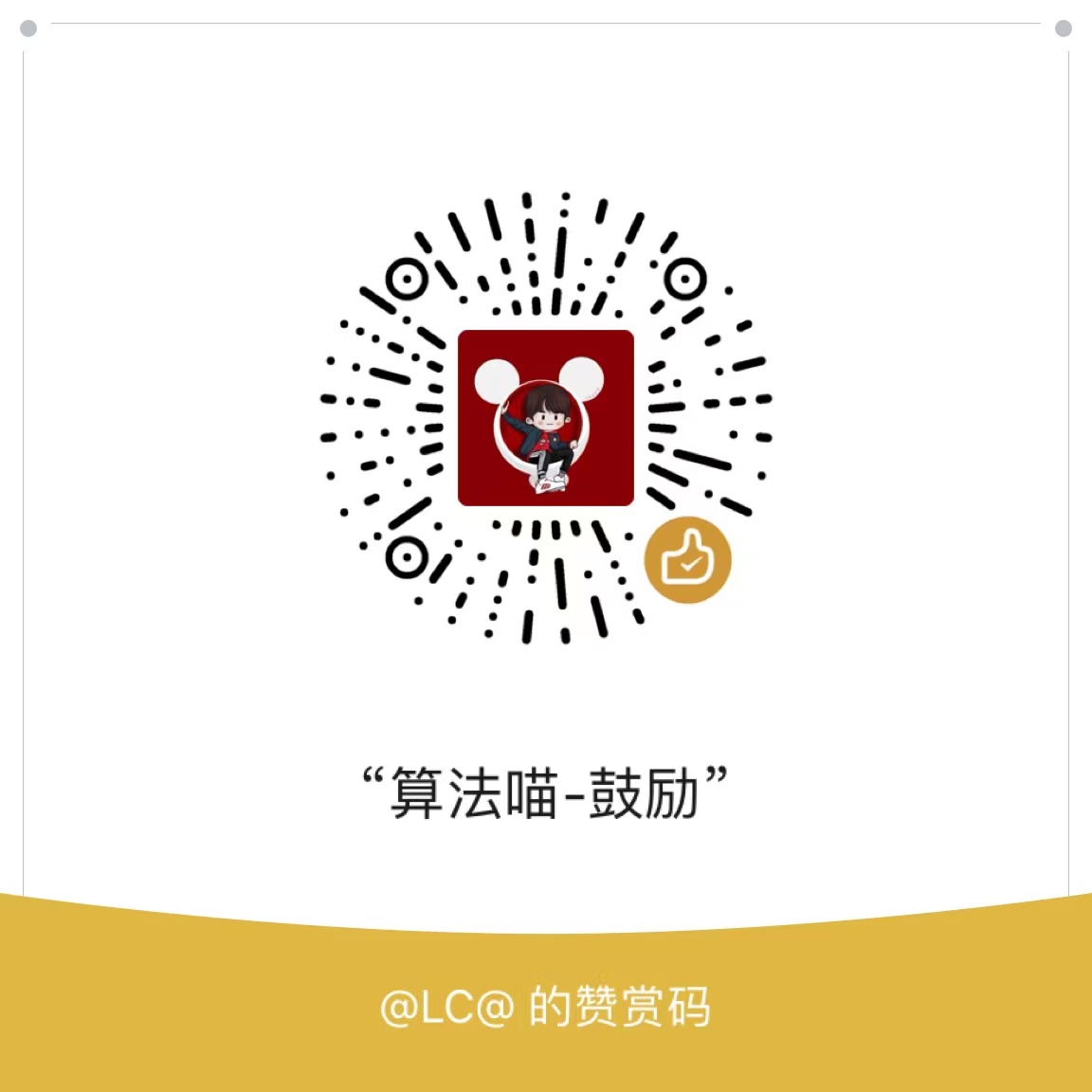