共计 4437 个字符,预计需要花费 12 分钟才能阅读完成。
Series is a one-dimensional labeled array capable of holding any data type (integers, strings, floating point numbers, Python objects, etc.). The axis labels are collectively referred to as the index. The basic method to create a Series is to call:
Series 是一维标记数组,能够包含任意类型数据
>>> s = pd.Series(data, index=index)
Here, data can be many different things:
- a Python dict
- an ndarray
- a scalar value (like 5)
数据可以是python字典或者n维数组或者一个常数值
The passed index is a list of axis labels. Thus, this separates into a few cases depending on what data is: From ndarray
index是series数据的索引,index值得确定要根据不同的数据来源以及定义
If data is an ndarray, index must be the same length as data. If no index is passed, one will be created having values [0, …, len(data) – 1].
数据来源数组
如果数据是n维数组,那么index的长度与数据的长度一致,如果没有定义index则会自增产生相应数据【0 len(data)-1】
In [1]: import numpy as np
In [2]: import pandas as pd
In [3]: s = pd.Series(np.random.randn(5), index=[‘a’, ‘b’, ‘c’, ‘d’, ‘e’])
In [4]: s
Out[4]:
a 0.2941
b 0.2869
c 1.70
d -0.2126
e 0.2696
dtype: float64
In [5]: s.index
Out[5]:
Index([‘a’, ‘b’, ‘c’, ‘d’, ‘e’], dtype=’object’)
In [6]: pd.Series(np.random.randn(5))
0 -0.4531
1 -1.8215
2 -0.1263
3 -0.1533
4 0.4055
dtype: float64
Note: Starting in v0.8.0, pandas supports non-unique index values. If an operation that does not support duplicate index values is attempted, an exception will be raised at that time. The reason for being lazy is nearly all performance- based (there are many instances in computations, like parts of GroupBy, where the index is not used).
From dict
数据来源字典
If data is a dict, if index is passed the values in data corresponding to the labels in the index will be pulled out.
Otherwise, an index will be constructed from the sorted keys of the dict, if possible.
index的构造来自字典的排序后的键值
Note: NaN(not a number)is the standard missing data marker used in pandas
From scalar value If data is a scalar value, an index must be provided. The value will be repeated to match the length of index
如果定义的index没有对应的值则使用nan来代替,始终要保证数据的长度与index的长度一致
In [7]: d = {‘a’ : 0., ‘b’ : 1., ‘c’ : 2.}
In [8]: pd.Series(d)
Out[8]:
a 0.0
b 1.0
c 2.0
dtype: float64
In [9]: pd.Series(d, index=[‘b’, ‘c’, ‘d’, ‘a’])
Out[9]:
b 1.0
c 2.0
d NaN
a 0.0
dtype: float64
In [10]: pd.Series(5., index=[‘a’, ‘b’, ‘c’, ‘d’, ‘e’])
Out[10]:
a 5.0
b 5.0
c 5.0
Series 类似于n维数组
Series acts very similarly to a ndarray, and is a valid argument to most NumPy functions. However, things like slicing also slice the index.
In [11]: s[0]
Out[11]: 0.29413876297575337
In [12]: s[:3]
Out[12]:
a 0.2941
b 0.2869
c 1.7098
dtype: float64
In [13]: s[s > s.median()]
Out[13]:
a 0.2941
c 1.7098
dtype: float64
In [14]: s[[4, 3, 1]]
e 0.2696
d -0.2126
b 0.2869
dtype: float64
In [15]: np.exp(s)
a 1.3420
b 1.3323
c 5.5276
d 0.8085
e 1.3094
dtype: float64
Series类似字典
A Series is like a fixed-size dict in that you can get and set values by index label:
In [16]: s[‘a’]
Out[16]: 0.29413876297575337
In [17]: s[‘e’] = 12.
In [18]: s
Out[18]:
a 0.2941
b 0.2869
c 1.7098
d -0.2126
e 12.0000
dtype: float64
In [19]: ‘e’ in s
Out[19]:
True
In [20]: ‘f’ in s
False
If a label is not contained, an exception is raised:
Using the get method, a missing label will return None or specified default:
See also the section on attribute access.
Series向量化操作
When doing data analysis, as with raw NumPy arrays looping through Series value-by-value is usually not necessary. Series can also be passed into most NumPy methods expecting an ndarray.
在做数据分析时,需要经常对数组中的每个值进行相应的操作,使用循环迭代的方式并不是想要的,series可以传递给numpy中的方法直接运算
In [23]: s + s
Out[23]:
0.2941
0.2869
1.7098
-0.2126
12.0000
In [21]: s.get(‘f’)
s[‘f’] KeyError: ‘f’
In [22]: s.get(‘f’, np.nan)
Out[22]: nan
A key difference between Series and ndarray is that operations between Series automatically align the data based on label. Thus, you can write computations without giving consideration to whether the Series involved have the same labels.
series与ndarray的区别在于series会自动按照标签对齐数据,所以在进行运算的时候不需要担心数据不是相同的标签
The result of an operation between unaligned Series will have the union of the indexes involved. If a label is not found in one Series or the other, the result will be marked as missing NaN. Being able to write code without doing any explicit data alignment grants immense freedom and flexibility in interactive data analysis and research. The integrated data alignment features of the pandas data structures set pandas apart from the majority of related tools for working with labeled data.
计算的结果是会取两个series的标签的并集,没有标签的数据自动使用nan补全
Note: In general, we chose to make the default result of operations between differently indexed objects yield the union of the indexes in order to avoid loss of information. Having an index label, though the data is missing, is typically important information as part of a computation. You of course have the option of dropping labels with missing data via the dropna function.
Name attribute
name属性
Series can also have a name attribute:
In [27]: s = pd.Series(np.random.randn(5), name=‘something’)
In [28]: s
Out[28]:
0 -0.181904
1 -1.087438
2 0.432270
3 1.408268
4 0.123985
Name: something, dtype: float64
In [29]: s.name
‘something’
In [26]: s[1:] + s[:–1]
Out[26]:
a NAN
b 0.5739
c 3.4195
d -0.4252
e NAN
dtype: float64
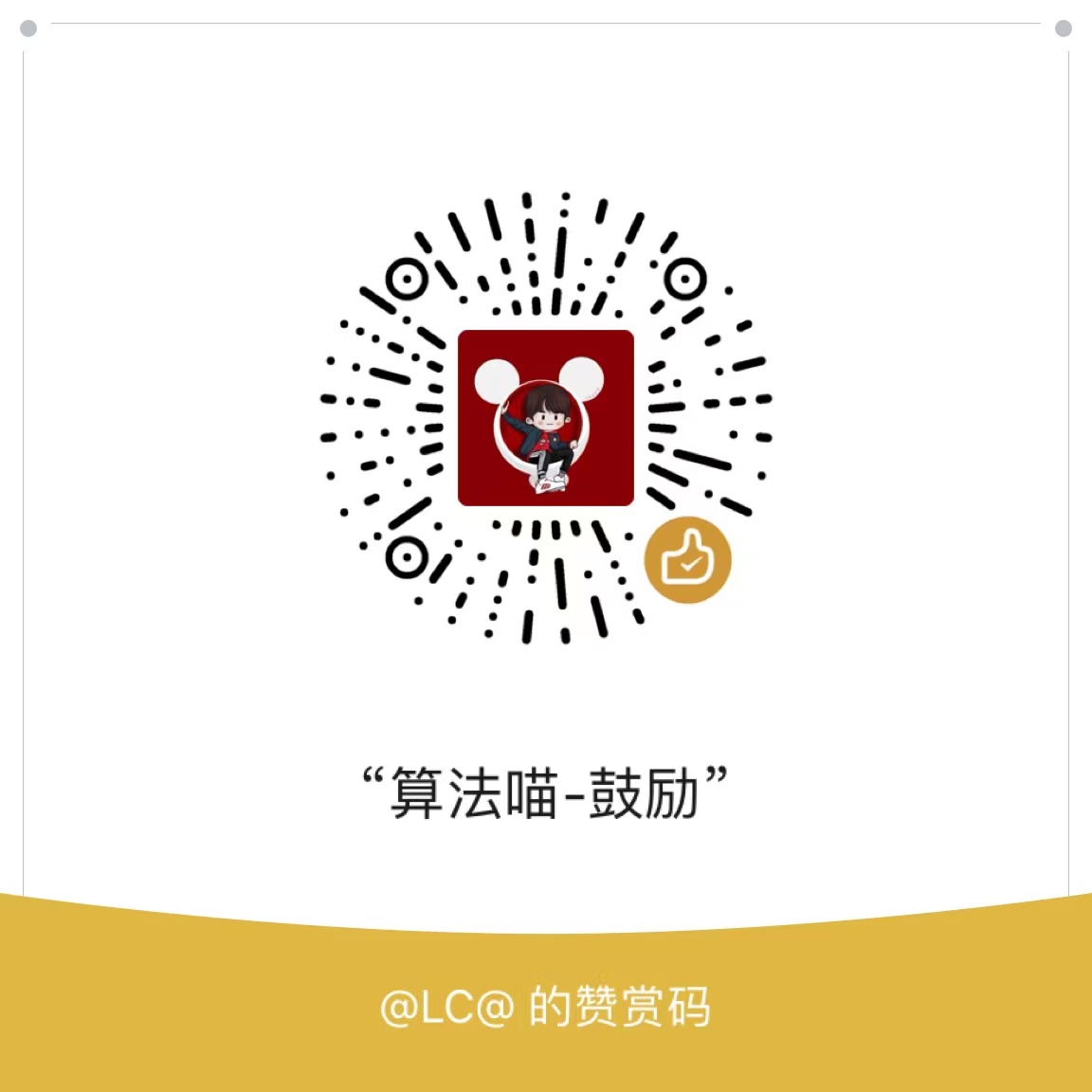